Sentiment Associations of Politically Loaded Terms in News Media
Summary of manuscript “Using Word Embeddings to Probe Sentiment Associations of Politically Loaded Terms in News and Opinion Articles from News Media Outlets”
Published article: Using Word Embeddings to Probe Sentiment Associations of Politically Loaded Terms in News and Opinion Articles from News Media Outlets
Introduction
I recently published an article with Musa al-Gharbi where we describe an analysis of political associations in 27 million diachronic (1975-2019) news and opinion articles from 47 news media outlets popular in the United States. We used word2vec embedding models trained on individual outlets content to quantify temporal and outlet-specific latent associations between positive/negative sentiment words and terms loaded with political connotations such as those describing political orientation, party affiliation, names of influential politicians and ideologically aligned public figures.
Word embedding algorithms are a language modelling technique that capture semantic associations between words within a text corpora into dense vector representations [1]. Others have previously used word embeddings to track the temporal evolution of cultural associations and stereotypes around the dimensions of gender and ethnicity [2] as well as social class [3] in textual artifacts. It has also been noted that the associations discovered by diachronic word embeddings correlate significantly with associations expressed by individuals both in historical and contemporary surveys [3]. Thus, a word embedding model trained on a specific corpus produced within a given cultural system can serve as a useful proxy to elucidate the idiosyncratic conscious and unconscious associations held or used by the group that produced the corpus of text.
Embedding spaces capture quantitative associations about the empirical world
We replicated previous results [4] about gender associations in popular pre-trained embedding models by constructing a gender axis in an embedding model trained on New York Times articles written between 2015-2019. This is done by averaging into a vector representation a set of male denoting words (i.e. man, men, male, males) and substracting the result from an aggregate vector of female denoting words (i.e., woman, women, female, females) to create the gender axis. We then project terms describing professions onto the gender axis and correlate the landing locations of the profession terms projections onto the gender axis with the percentage of female representation in each profession. The results show that professions with a high percentage of female representation tend to project towards the female pole of the gender axis and male-dominated professions tend to project towards the masculine pole of the gender axis. Thus, mimicking the statistical relationship between professions and their percentage of female representation in the job market, see Figure 1. We use similar methodology to also show that the embedding space derived from news articles also captures properties about countries economic development, car manufacturer brand prices and political affiliation of U.S. senators, see Figure 1B-C.
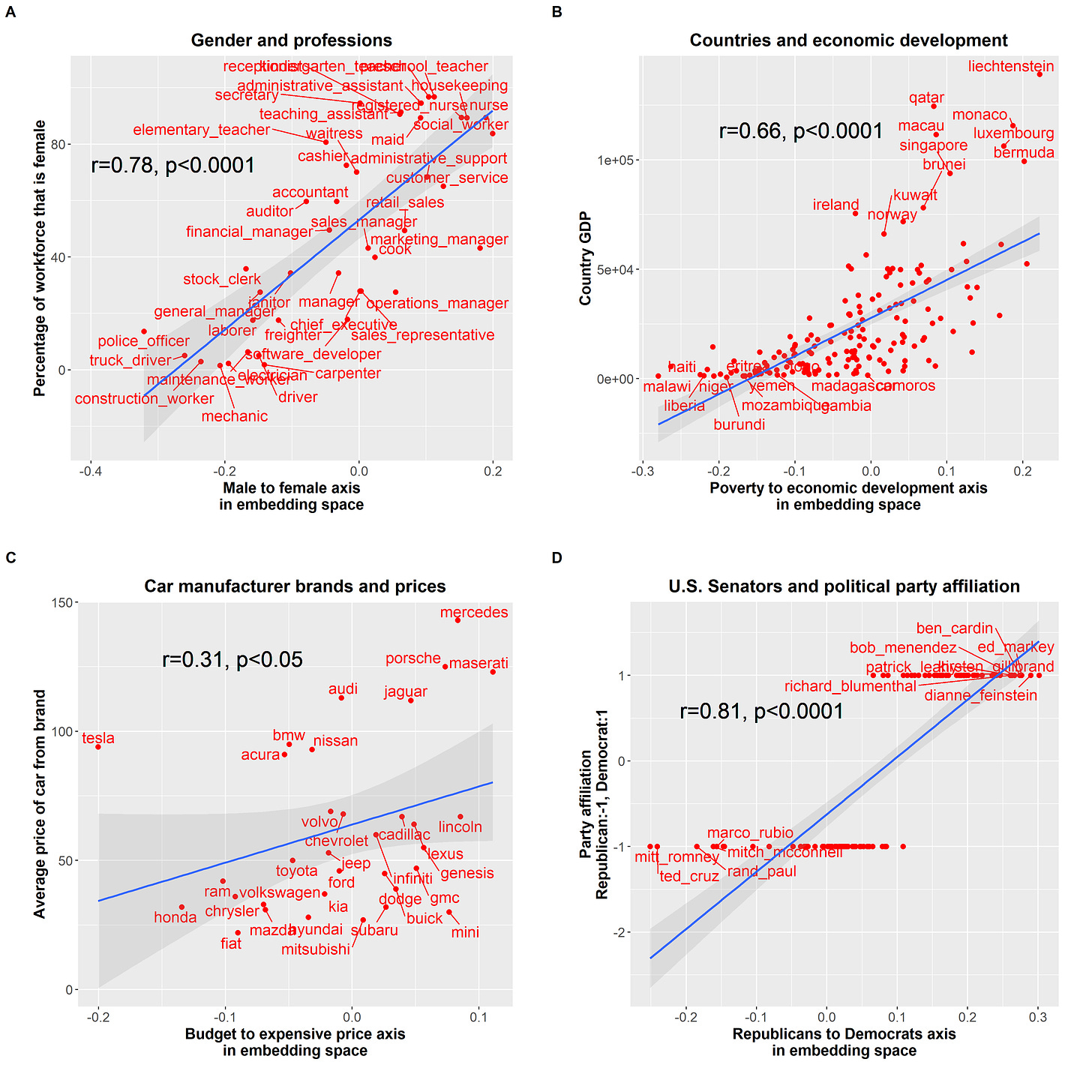
Projections of sentiment lexicons onto cultural axes with widespread accepted connotations of positivity and negativity
To validate that embedding spaces derived from news outlets content also capture many of the intuitive sentiment associations held by humans, four cultural axes were created in the same word embedding model derived from New York Times articles used in Figure 1. The four cultural axes are Death-Life, Disease-Health, Dictatorship-Democracy and malevolent to respectable historical figures. When we project onto these axes a sentiment lexicon, positive words tend to be associated with the poles denoting life, health, democracy and widely admired historical figures (such as Martin Luther King, Gandhi or Nelson Mandela), see Figure 2. Negative words tend to be associated with the poles denoting death, disease, dictatorship and malevolent historical figures (such as Hitler or Stalin) see Figure 2. These results suggest that word embedding models trained on news outlet content capture many of the intuitive positive and negative sentiment associations held by humans. Thus, it is reasonable to use this technique to measure prevalent sentiment associations with respect to political orientation in embedding models derived from news outlets textual content.
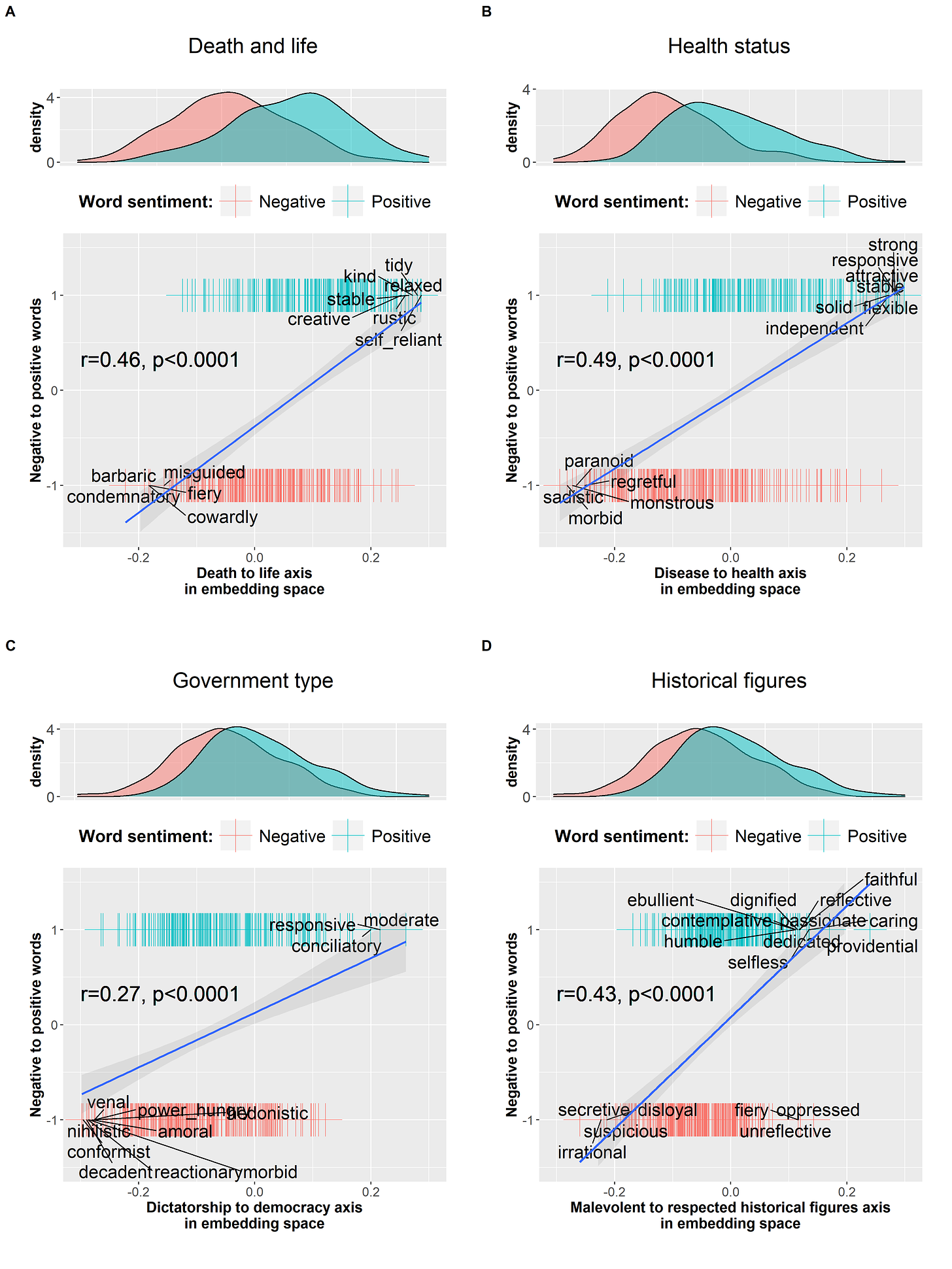
Building cultural axes representative of political orientation
We built six axes representing political orientation for analysis purposes by assigning terms to the poles of the axis based on ideological affiliation. A personal ideology cultural axis is built with terms such as conservative, right-winger, or right-leaning forming the conservative pole of the axis and terms such as liberal, left-winger or left-leaning forming the liberal pole of the axis. The party affiliation cultural axis is built using terms such as Republicans, RepublicanParty and Democrats, DemocraticParty, see Figure 3. The U.S. presidents’ cultural axis is built with the names of all U.S. presidents since World War II. The axis of ideologically oriented journalists is built using an external list from Politico [5] containing 35 influential journalists such as Anderson Cooper, Rachel Maddow, Sean Hannity or Tucker Carlson. The U.S. senators axis is built with the names of all Republican and Democratic senators in the U.S. Senate as of March 2020. Finally, the influential conservatives and liberals axis is built using two external lists from The Telegraph newspaper that ranked influential liberal [6] and conservative [7] public figures in the U.S. such as Supreme Court justices, high-ranking administration officials, politicians and journalists.
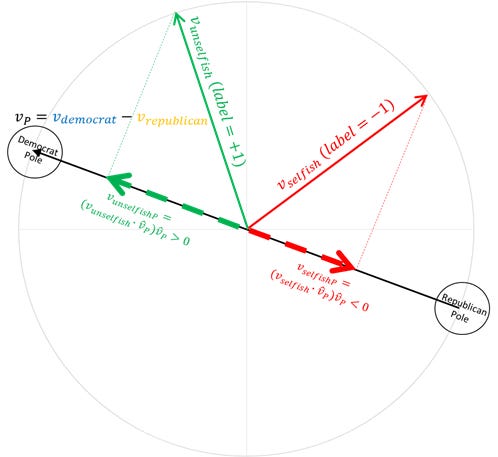
Political associations in outlet-specific embedding models and external human ratings of outlets ideological bias
To measure political associations in news media outlets, we trained word2vec embedding models on outlet-specific news and opinion articles (2015-2019) and quantified the degree of correlation between sentiment words labels (positive and negative) and the projection values of said sentiment words onto axes tracing the spectrum of political orientation in the embedding models representative of each outlet. A positive value in the Y-axis of Figure 4 signifies associations favorable to left-wing denoting terms. A negative value signifies associations favorable to right-wing denoting terms.
Right-leaning news outlets tend to associate negative terms with liberals, Democrats, and left-wing public figures and positive terms with conservatives, Republicans and right-wing public figures. An inverse association is observable in left-leaning news outlets. Outlets ranked as centrist by humans (see AllSides [8]) display associations that are milder but more similar in direction to those of left-leaning outlets than those of right-leaning media.
Weighting the associations of the top five most popular outlets in each political orientation category by monthly visitors to their online domains, according to web traffic metrics from SimilarWeb.com, we obtain a weighted average of written news media political associations reach into society (dashed black horizontal lines in Figure 3). This metric appears to consistently show a larger reach of left-leaning media associations into society than right-leaning ones for most political axes.
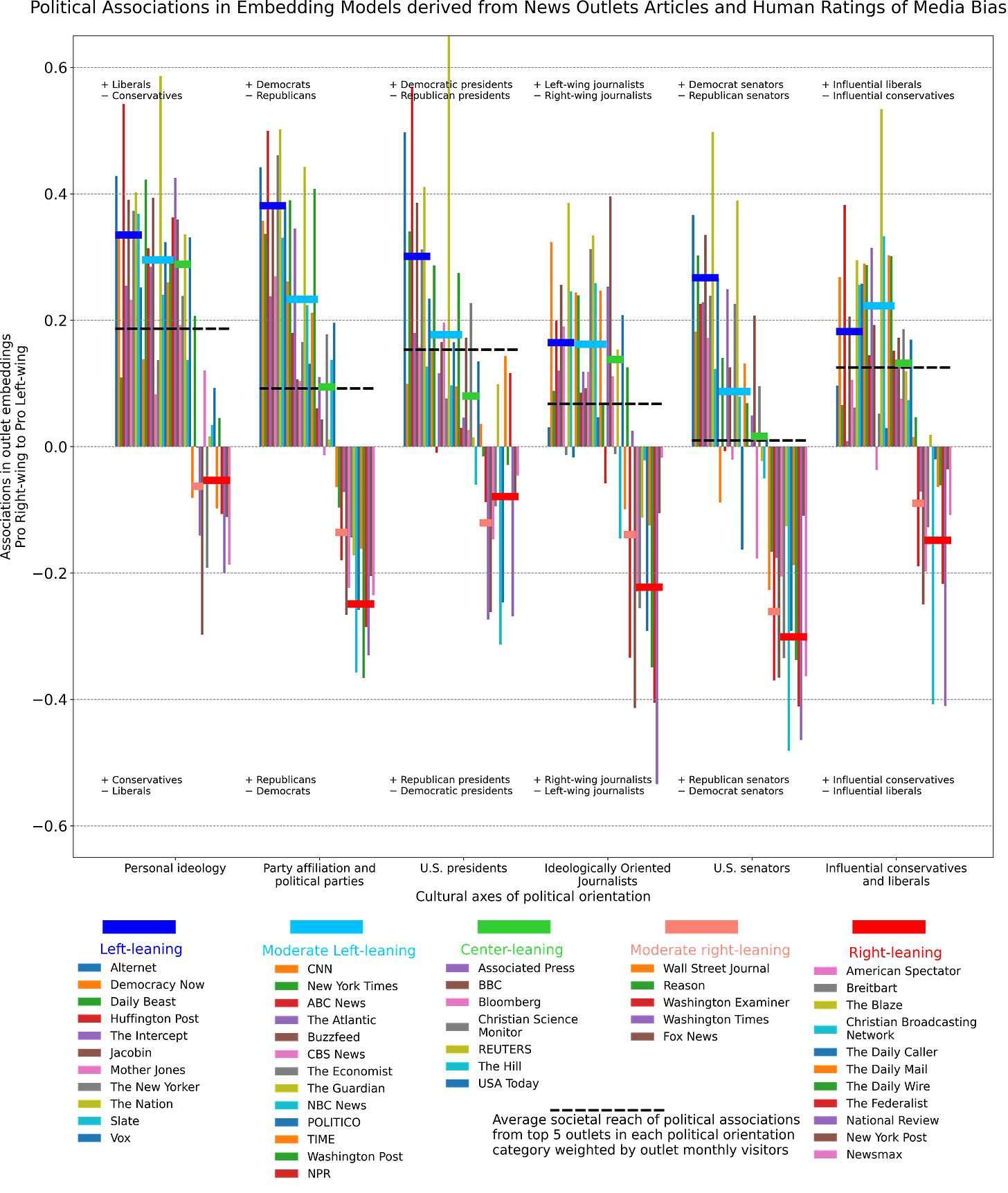
Pearson’s r correlations between political associations in outlet-specific embedding models (Y-axis in Figure 4) and external human ratings of outlet ideological bias (AllSides [8]) were substantial regardless of political axis analysed (see scatter plots in Figure 5 ). The results described above are consistent regardless of sentiment lexicon or human ratings of media bias used.
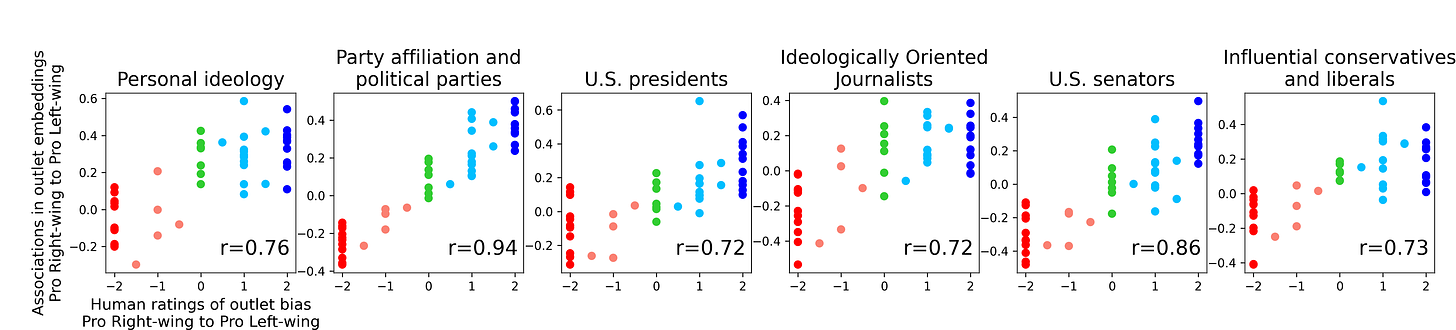
A comprehensive visualization of the political orientation sentiment associations displayed by influential media outlets across 2 different political axes is shown in Figure 6. Positive (in cyan) and negative (in salmon) words from 19 external sentiment lexicons (N=15,704) are projected onto 2 political orientation axes of the seven most visited news media outlets in each broadly defined ideological orientation partition: left-leaning (top row), centrist (middle row) and right-leaning (bottom row). A clear tendency of left-leaning media outlets to associate liberals and Democrats with positive words (upper right quadrant of the plane) and conversely conservatives and Republicans with negative words (lower left quadrant of the plane) is apparent. A similar but milder trend is also apparent in some human rated centrist outlets. A few outlets display what appears to be mild or neutral associations. Several right-leaning media outlets display an opposite trend to left-leaning media outlets: a tendency to associate negative terms with liberals and Democrats and positive terms with conservatives and Republicans.
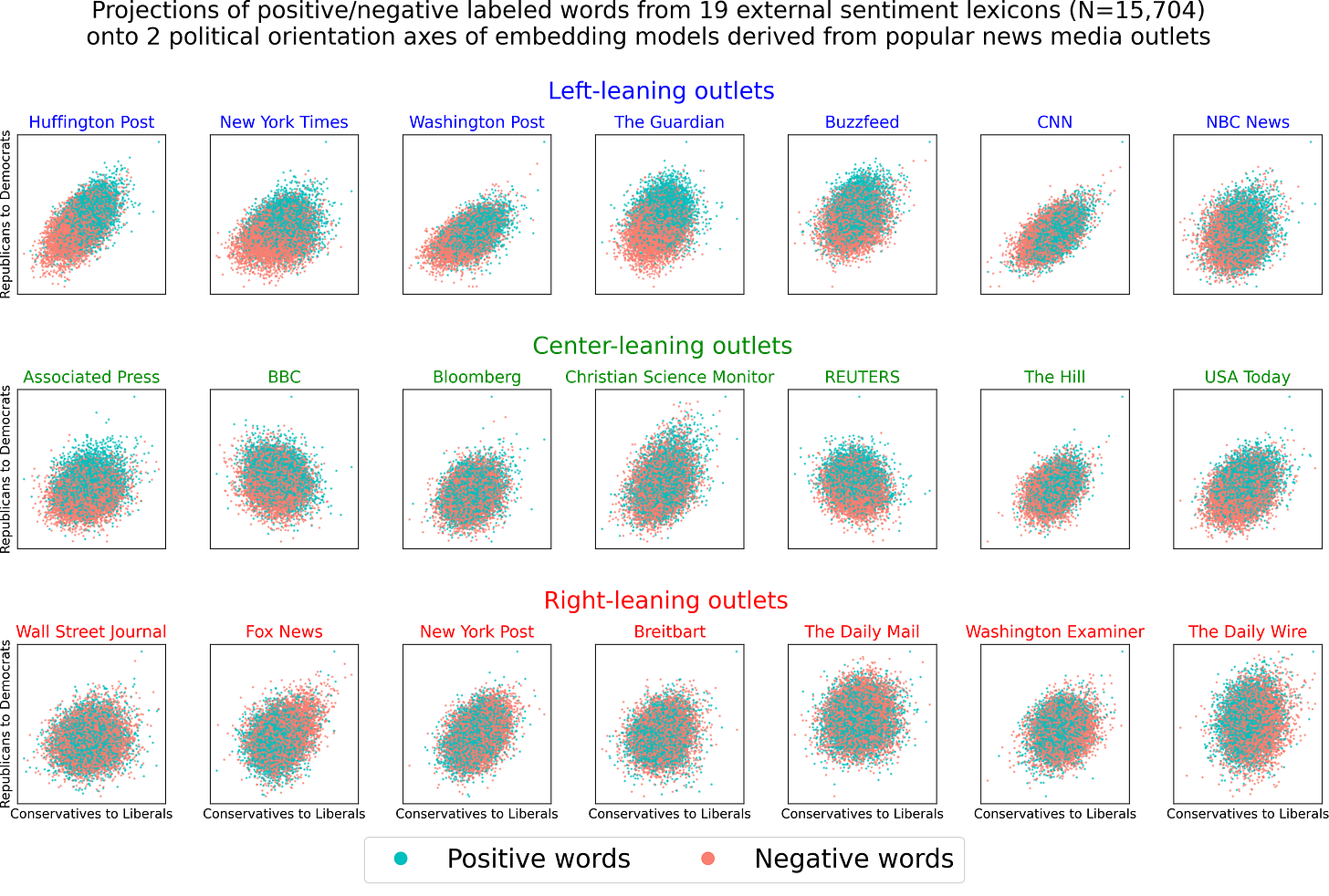
Comparing news media political associations between 2010-2014 and 2015-2019
The aforementioned results were measured in news and opinion articles from the 2015-2019 time range. In order to test whether outlets’ associations are stable over time or instead reflect epiphenomena idiosyncratic to the socio-political moment between 2015 and 2019, we next compared political associations in media outlets for word embedding models trained on news outlets content from the 2015-2019 time interval versus embedding models trained on news outlets content from the 2010-2014 time range, see Figure 7. The results appear to display mild evidence of growing polarization across political axes. However, associations in 2010-2014 content display similar political leanings as those between 2015 and 2019, as evidenced by consistently larger political sentiment association values in the y-axes for left-leaning (blue) outlets, denoting left-leaning preferential associations, than right-leaning (red) outlets. This suggests that the specific idiosyncrasies of the sociopolitical environment in the 2015-2019 time period have not been the only factor shaping the political sentiment associations described in Figures 4, 5 and 6.
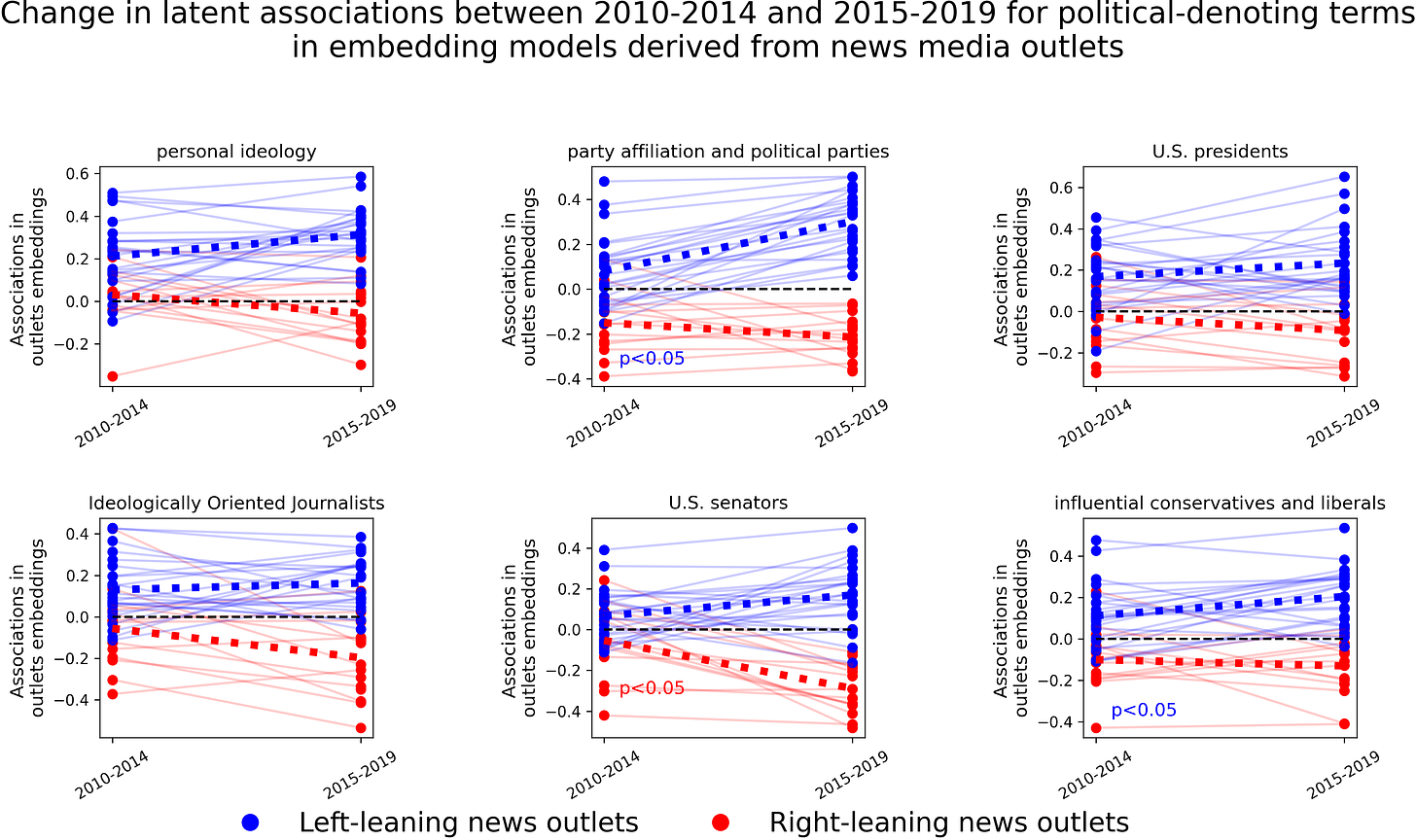
Long-term temporal dynamics of political associations in news media content
Figure 8 displays sentiment associations dynamics from 1975 to 2019, measured using outlets’ content at 5-year intervals. Representative online news articles availability for right-leaning outlets only starts in 1996. Only three political axes formed with terms that have been fairly prevalent since the 1970s, such as conservatives, liberals, Republicans or names of U.S. presidents since World War II were analyzed. The dashed horizontal black line in the middle of each plot reveals the location of neutral association in embedding space. An association of negative sentiment terms with Pole 1 and/or positive sentiment terms with Pole 2 of an axis, results in a positive correlation (above the dotted black line). Conversely, an association of negative sentiment terms with Pole 2 and/or positive sentiment terms with Pole 1 of an axis, results in a negative correlation (below the dotted red line).
Across the three ideological orientation axes analyzed, left-leaning outlets appear to have become more partisan over time and increasingly associate positive terms with words that denote their own ideological tribe and negative terms with words that denote their ideological outgroup. Right-leaning news outlets also appear to have grown more polarized at least for some of the axes analyzed.
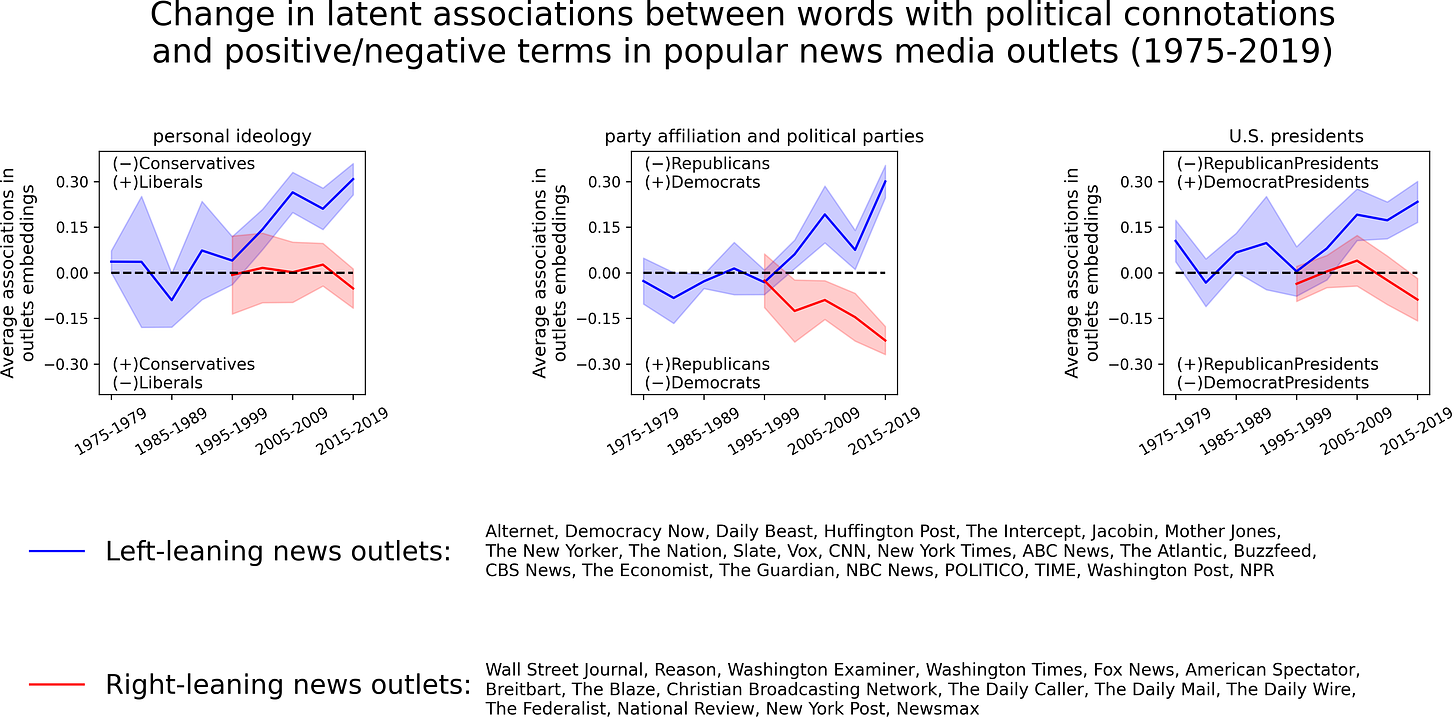
Conclusion
Our results show that word embeddings algorithms can be used to computationally measure political sentiment associations in news media content and that such measurements correlate substantially with human perceptions of news media political bias (r>0.7). Another relevant finding has been that political associations in news media articles appear to display growing polarization with respect to how partisan media portray members of the ‘other’ faction.
Examining Figures 4, 5 and 8 could lead to infer that political bias in left of center news media outlets is more acute than in right of center news outlets. Although this could conceivably be the case, it is not necessarily so. The inclusion of newswires from news agencies such as Associated Press or Reuters, that appear to display mild left-leaning associations (see Figure 6), in both right and left leaning media outlets, creates a confounding factor that could be ameliorating the pro right-leaning bias embedded in right of center outlets own content.
A fundamental limitation of this work is that it is not clear how to precisely locate the neutral point of unbiased news. As highlighted previously by others [13], we lack a ground truth of neutrally covered real world events that we could use as a reference to estimate unbiased news content. While the aggregate political association sentiment measures are validated by their high degree of correlation with human ratings of news outlets political bias, specific associations can sometimes be challenging to interpret. Beyond political animosity towards the ideological outgroup, other causal roots could be at play in creating such associations such as a particular group using more negative language or producing a lot of detrimental news for themselves.
An important question arising from this work is what are the social consequences of news media bias and the increasing political polarization of news media content. On the one hand, Americans trust in news media appears to be eroding [9], with this pattern being particularly acute among Republicans [10]. While most people would agree that fair and unbiased news reporting is an important element of a healthy civic culture, the commercial success of allegedly partisan news outlets suggests that there is a substantial market appetite for news content that supports the viewership’s political predilections [11], [12]. The societal effects of increasingly partisan news media supply and consumption is therefore a relevant topic for future research.
References
[1] T. Mikolov, I. Sutskever, K. Chen, G. S. Corrado, and J. Dean, “Distributed Representations of Words and Phrases and their Compositionality,” in Advances in Neural Information Processing Systems 26, C. J. C. Burges, L. Bottou, M. Welling, Z. Ghahramani, and K. Q. Weinberger, Eds. Curran Associates, Inc., 2013, pp. 3111–3119. Accessed: May 11, 2017. [Online]. Available: http://papers.nips.cc/paper/5021-distributed-representations-of-words-and-phrases-and-their-compositionality.pdf
[2] N. Garg, L. Schiebinger, D. Jurafsky, and J. Zou, “Word embeddings quantify 100 years of gender and ethnic stereotypes,” Proc. Natl. Acad. Sci., vol. 115, no. 16, pp. E3635–E3644, Apr. 2018, doi: 10.1073/pnas.1720347115.
[3] A. C. Kozlowski, M. Taddy, and J. A. Evans, “The Geometry of Culture: Analyzing the Meanings of Class through Word Embeddings,” Am. Sociol. Rev., vol. 84, no. 5, pp. 905–949, Oct. 2019, doi: 10.1177/0003122419877135.
[4] A. Caliskan, J. J. Bryson, and A. Narayanan, “Semantics derived automatically from language corpora contain human-like biases,” Science, vol. 356, no. 6334, pp. 183–186, Apr. 2017, doi: 10.1126/science.aal4230.
[5] D. Byers, “Twitter’s most influential political journalists,” POLITICO. https://www.politico.com/blogs/media/2015/04/twitters-most-influential-political-journalists-205510.html (accessed Aug. 02, 2020).
[6] T. Harnden, “The most influential US liberals: 20-1,” Jan. 15, 2010. Accessed: Aug. 02, 2020. [Online]. Available: https://www.telegraph.co.uk/news/worldnews/northamerica/usa/6991000/The-most-influential-US-liberals-20-1.html
[7] T. Harnden, “The most influential US conservatives: 20-1,” Jan. 15, 2010. Accessed: Aug. 02, 2020. [Online]. Available: https://www.telegraph.co.uk/news/worldnews/northamerica/usa/6990965/The-most-influential-US-conservatives-20-1.html
[8] “AllSides Media Bias Ratings,” AllSides, 2019. https://www.allsides.com/blog/updated-allsides-media-bias-chart-version-11 (accessed May 10, 2020).
[9] G. Inc, “Americans’ Trust in Mass Media Sinks to New Low,” Gallup.com, Sep. 14, 2016. https://news.gallup.com/poll/195542/americans-trust-mass-media-sinks-new-low.aspx (accessed May 06, 2021).
[10] G. Inc, “Americans Remain Distrustful of Mass Media,” Gallup.com, Sep. 30, 2020. https://news.gallup.com/poll/321116/americans-remain-distrustful-mass-media.aspx (accessed May 06, 2021).
[11] M. al-Gharbi, “The New York Times’ obsession with Trump, quantified,” Columbia Journalism Review. https://www.cjr.org/covering_the_election/new-york-times-trump.php (accessed May 09, 2020).
[12] M. al-Gharbi, “Cable news profits from its obsession with Trump. Viewers are the only victims.,” Columbia Journalism Review, Sep. 08, 2020. https://www.cjr.org/politics/cable-news-trump-obsession.php (accessed Mar. 22, 2021).