Is Wikipedia Politically Biased?
A computational analysis of Wikipedia's content suggests that Wikipedia’s Neutral Point of View policy is not achieving its stated goal of impartiality
I have recently published an analysis attempting to determine whether there is evidence of political bias in English Wikipedia articles. As a supporter of Wikipedia’s mission, I view the findings as a call to refine the platform's content, not undermine its value.
To study political bias in Wikipedia content, I first gathered from external sources a set of target terms (N=1,628) with political connotations (i.e. names of recent U.S. presidents, U.S. congressmembers, U.S. Supreme Court Justices or Prime Ministers of Western countries). I did not cherry pick the set of terms to be included in the analysis but instead used publicly available pre-existing lists of terms from Wikipedia and other sources. I then identified all mentions in English Wikipedia of those terms. This was followed by extraction of the textual context around those terms and feeding a random sample of those text snippets to a machine learning model for annotation of the sentiment/emotion with which the target term is used in the snippet (in total, I generated 175,205 sentiment annotations).
Results show a mild to moderate tendency in Wikipedia articles to associate public figures ideologically aligned right-of-center with more negative sentiment than public figures ideologically aligned left-of-center. These results suggest that Wikipedia’s neutral point of view policy (NPOV) is not achieving its stated goal of viewpoint impartiality in Wikipedia articles.
The following figure shows the average sentiment with which U.S. presidents, U.S. senators, U.S. House of Representatives Congressmembers from the 117th Congress and U.S. State Governors (as of 2022) are used in Wikipedia articles. There is an average tendency in Wikipedia articles to use the names of prominent left-leaning U.S. politicians with more positive sentiment than their right-leaning counterparts.
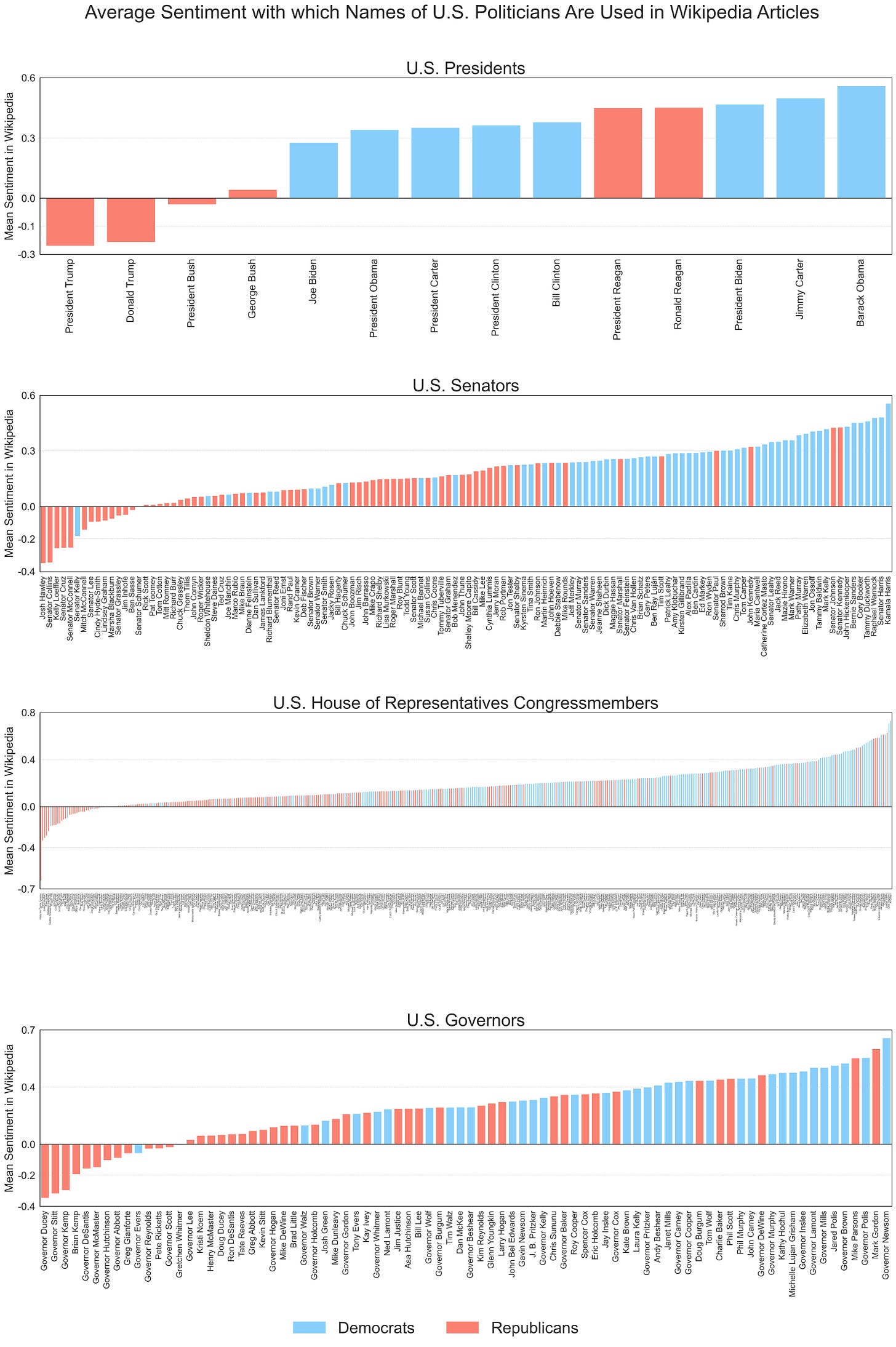
The tendency in Wikipedia articles for names of prominent left-leaning politicians to be used, on average, with more positive sentiment than their right-leaning counterparts is not circumscribed to political figures. Similar associations are also apparent when examining the average sentiment with which the names of U.S. Supreme Court Justices and U.S. based journalists are used in Wikipedia articles
The phenomenon described above is not circumscribed to American public figures. A similar trend is also observable in the way that Prime Ministers (since the year 2000) from prominent Western countries are used in Wikipedia articles. This pattern however is not apparent when examining Wikipedia mentions of U.K. MPs. Note that the dominant red color in the bottom of the Figure below is due to the larger number of Conservative MPs than labor MPs in the U.K. parliament as a result of the 2019 United Kingdom general election. It should be noted that Wikipedia entries about non-political public figures sharing the same name with political figures could be playing a confounding role in this regard.
The asymmetric sentiment with which politically aligned public figures are used in Wikipedia content also seems to occasionally extend to some organizations and institutions. There is a tendency in Wikipedia articles to associate left-leaning news media institutions with more positive sentiment than right-leaning news media organizations. This asymmetry however is not apparent for mentions in Wikipedia of U.S. based think tanks.
I summarize the trends above and a few others in a single figure while also applying statistical t-tests for each category of terms to determine if the difference in sentiment with which left-leaning and right-leaning terms are used in Wikipedia articles is statistically significant, see Figure below. I mark with an asterisk each category of results that are statistically significant at the 0.01 threshold. However, since the number of terms in each category is vastly different and the p-values are dependent on the sample size of each test, said p-values are not informative as to assess the magnitude of the difference between the two groups. Hence, I also provide an estimate of effect size (Cohen’s d) for the difference between the two groups in each test. For most of the categories analyzed, there are substantial effect sizes for the differences in the sentiment with which right-leaning and left-leaning terms are used in Wikipedia articles. The t-test for U.K. MPs is borderline significant but the effect size is negligible.
I next inspect the average emotion with which the examined target terms are used in Wikipedia articles. I use Ekman’s 6 basic emotions (anger, disgust, fear, joy, sadness, and surprise) plus neutral to annotate the emotions with which the set of target terms with political connotations are used in Wikipedia content. For ease of visualization, I exclude the emotionally neutral category in the next visualization. In the Figure below, it can be observed that in Wikipedia articles right-leaning terms are more often associated with the emotional categories of anger and disgust than left-leaning terms. In contrast, left-leaning terms are more often associated with the emotion of joy than right-leaning terms.
The findings above should be viewed constructively, highlighting the inherent challenges of maintaining neutrality in a user-generated content platform. Recognizing these challenges is essential for developing strategies to enhance content impartiality. Wikipedia's editors could benefit from better tools to identify and reduce bias. Computational tools to detect biased content and adversarial collaborative mechanisms like X’s Community Notes could aid in this process. While acknowledging Wikipedia's immense contributions to open knowledge, the results of my analysis suggest that Wikipedia’s journey towards complete impartiality is ongoing.
Given that Wikipedia content is routinely used to train Large Language Models (LLMs)—the engines driving many cutting-edge AI systems such as ChatGPT—the biases observed in Wikipedia could infiltrate into the parameters of AI models, thereby propagating said biases further through AI generated content. In fact, I also found a mild to moderate positive correlation between prevailing sentiment polarity political associations in word embeddings derived directly from Wikipedia content and OpenAI embeddings. This suggests (though not conclusively) a potential transfer of Wikipedia biases into Artificial Intelligence models. I describe this set of results next.